Farm scale Climate Risk and Vulnerability Analysis
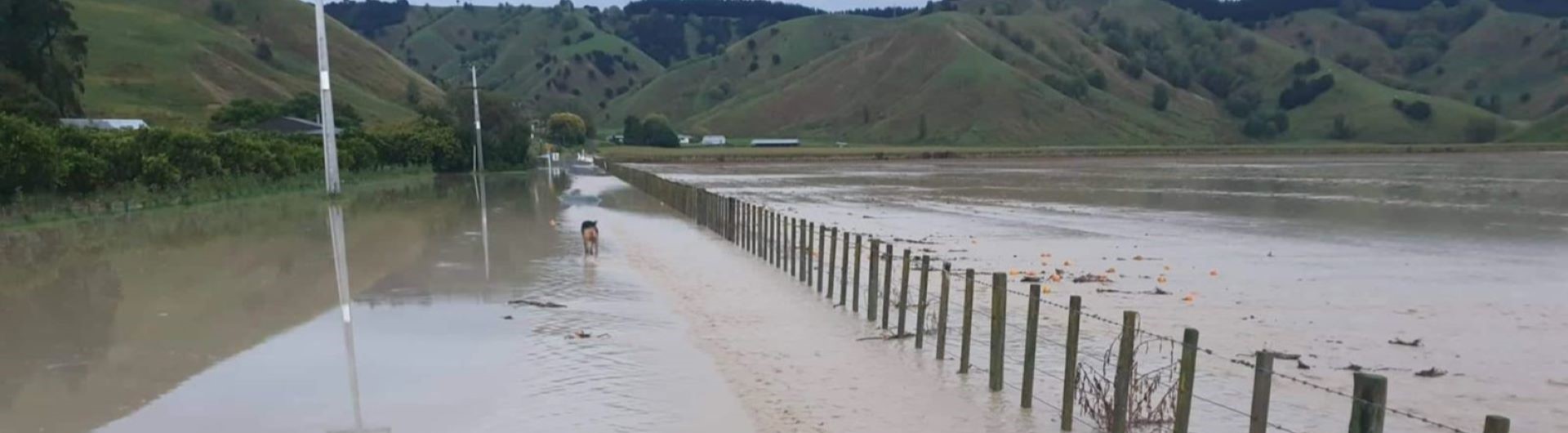
A climate hazard is a large-scale change in the climate system that takes place over a few decades and causes substantial disruptions to the farming system.
Prism has developed a climate risk and vulnerability method that has been used on Sheep and Beef, Venison, and Dairy farms across Aotearoa New Zealand. Our approach evaluates the climate hazards, vulnerability, and risk at a farm level at decadal intervals out to 2050 using an adapted Climate Risk Vulnerability assessment (CRVA) method. A CRVA identifies factors that contribute to vulnerability and risk, which can include both the direct and indirect effects of climate change. Underpinning our analysis is a set of digital data layers describing the farms natural resources including: soils and geology, water availability, slope and topography, land environment and land use capability, vegetative cover, erosion risk, mapped landslides, wind exposure, and more. Infrastructure data including roads, dams, ponds and more are then added.
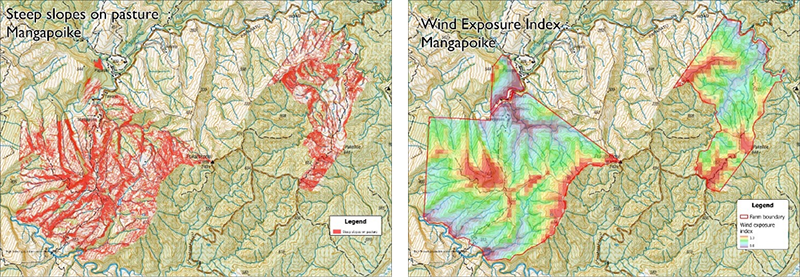
Climatic hazards are then examined. Firstly, the present-day climate is characterised based on weather data history. If a weather station exists on the farm the data is based on that, while elsewhere data is derived from NIWA virtual stations. The climate history is then assessed in context of climate change projections and regional models leading to a discussion about the expected hazards and gradual change. Hazards across six areas are assessed including: drought, extreme heat, extreme rain/flooding, fire, extreme cold and extreme wind. This work is led by New Zealand experts in extreme weather under climate change Prof D Frame and Dr L Harrington. An example 50+ year time series for rainfall and temperature from a farm weather station is shown below.

The expert judgements used in this analysis replace regional council or AgResearch climate report summaries for three reasons: the way (councils) have chosen their metrics of future climate change are often not impact-relevant, the uncertainty across ensemble models can be large, and their inability to ground-truth model projections against actual (farm) station observations also adds to the uncertainty.
Overall, this project uses more localised observational data and a clear understanding of where the uncertainties in dynamical downscaling frameworks come from. We use several indices including a rain volatility index to profile a farm on a national scale.
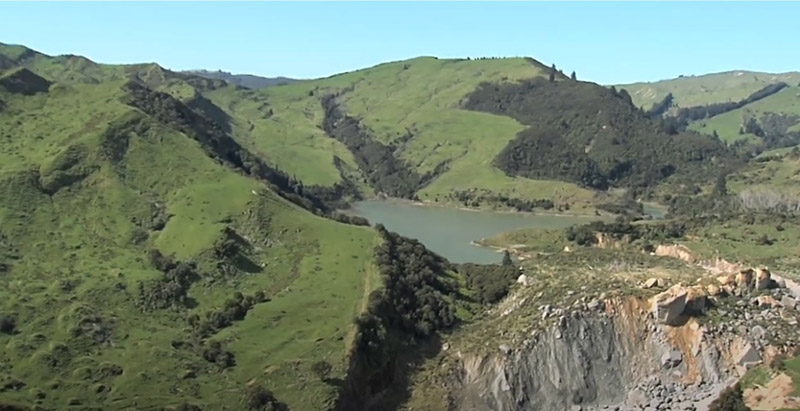
Landslide at Mangapoike, Tairawhiti during Cyclone Gabrielle. Photo Dan Jex Blake
Economic data from the farm is then examined in the context of weather-related stress events. Revenue, expenditure and EBIT are reviewed alongside stock units and production data. Finally the vulnerability to climate hazard over the period from present-day to 2050 is then identified noting that vulnerability refers to the predisposition of the farm to be adversely affected by climate hazards and encompasses farm capacity to cope and adapt.
Uncertainty is captured mathematically by the concept of probability with a quintile classification method. The product of hazard and vulnerability scores is indicative of the overall risk of a given hazard. Final climate vulnerability information is presented using a summary dashboard. A farm can improve its resilience and adaptive capacity to climate-related shocks and stresses by implementing climate adaptation actions.
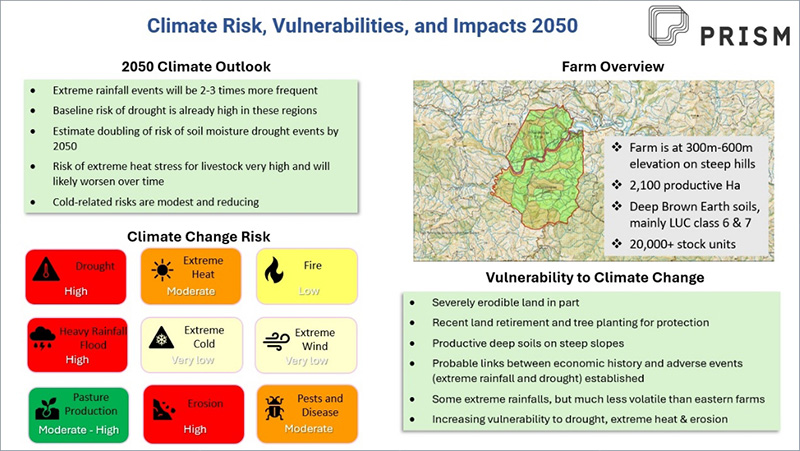